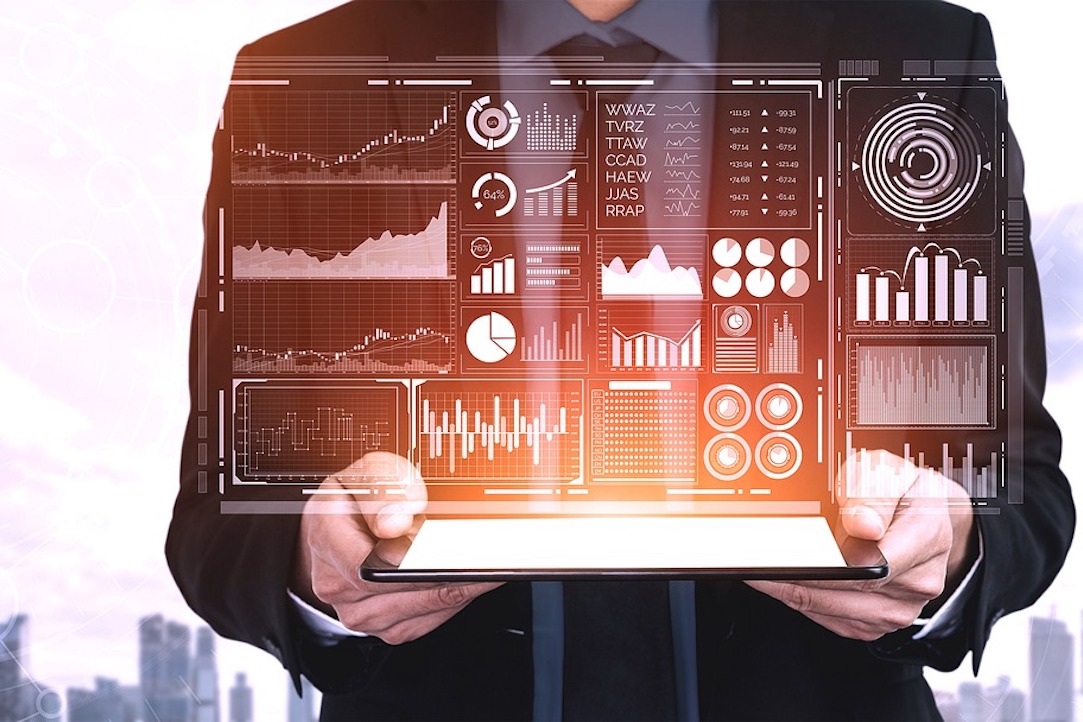
In today’s business environment, where risks continuously evolve and market dynamics constantly fluctuate, effective risk management is critical for financial institutions striving for stability and growth. Amidst such a challenging atmosphere, business intelligence (BI) tools have emerged as indispensable assets, revolutionizing the way risk analytics is conducted in the financial sector.
We will explore three fundamental capabilities of modern BI tools, such as Microsoft Power BI, SAP Business Objects, SAS Business Intelligence, and many others, that greatly enhance risk analytics, especially within the financial sector: data integration capabilities, data visualization capabilities, and real-time monitoring capabilities. Through practical examples, we’ll illustrate how these capabilities can empower banks and other financial institutions to make more informed decisions and mitigate risks effectively.
1) Data Integration Capabilities
Business Intelligence tools play a vital role in reshaping risk analytics practices in today’s world, primarily through their advanced data integration capabilities. By facilitating integration of diverse data sources (for example SQL databases, storage systems such as SharePoint and OneDrive, cloud-based platforms such as Azure and AWS, and various websites and other sources) these tools compile data from multiple sources, reducing manual input and enhancing risk assessment accuracy. This integration feature eliminates the need for complex and costly data integration solutions.
While Excel also allows data manipulation and basic integration, its capabilities are limited, making it less suitable for handling the complex and large amounts of data. With Excel, the user can import data from various sources such as CSV files, databases, or other spreadsheets and perform basic data transformations using functions such as filtering, sorting, and simple calculations. Excel, however, might not handle more complex data transformations and merging from multiple sources as effectively as business intelligence tools.
2) Data Visualization Capabilities
Visualization capabilities, in the context of business intelligence, refer to the transformation of complex datasets into advanced visual representations, including charts, graphs, and other depictions. Using advanced visualization capabilities of today’s BI tools, complex numerical data can be translated into a format that not only allows users to understand the meaning of numbers, but also helps decision-makers extract meaningful and actionable insights. Using customizable representations such as bar charts, candlestick charts, line graphs, scatter plots, pie charts, heat maps, treemaps, and geographic maps and others, risk analysts can display complex relationships and trends in a manner that is comprehensible to stakeholders at all levels and in dynamic formats. This interactive and dynamic approach can foster a deeper understanding of risk factors (such as market volatility) and their potential impacts.
In the context of visualization, advanced capabilities of BI tools go far beyond Excel. Their interactive and diverse visualizations, coupled with customization options, are tailored to render complex trends, patterns, and risk factors comprehensible. The financial sector, with its complex financial data and risk variables, can benefit from BI tools’ ability to transform numbers into actionable insights, whilst Excel’s basic chart types are better suited for simple analysis and visualizations.
3) Real-Time Monitoring Capabilities
In addition to data integration and visualization, another crucial capability of BI tools in risk analytics is real-time monitoring. With the fast-paced nature of financial markets and the ever-present threat of emerging risks, the ability to monitor and respond to changes in real-time is essential for effective risk management in the financial sector.
BI tools equipped with real-time monitoring capabilities allow banks to track key metrics and indicators continuously. This includes monitoring transaction flows, market prices, credit card usage patterns, and potential security breaches in real-time. By analyzing data as it is generated and detecting anomalies or deviations from expected patterns instantaneously, financial institutions can promptly identify and respond to emerging risks before they escalate into major issues.
While Excel is still used as a traditional tool for data analysis, its capabilities are limited when compared to BI tools, especially in terms of real-time monitoring for risk analytics. BI tools offer advanced features such as live data connectivity, automated alerts, and customizable dashboards, enabling banks to monitor key metrics continuously and respond to emerging risks and other red flags promptly.
Practical Examples from the Banking Industry
Example 1: ATM transaction Analysis and Monitoring
Let’s imagine a scenario where a bank’s risk management team utilizes a BI tool to analyze ATM transaction data to assess operational risks and optimize ATM usage. The data includes transaction logs from various ATMs across different branches, detailing the volume of transactions, withdrawal amounts, transaction times, and any instances of transaction failures or abnormalities.
With the aid of BI tools, the risk management team can swiftly connect to the bank’s database containing ATM transaction records. Using interactive dashboards and data visualization features, they can monitor key metrics such as transaction volume trends, peak transaction times, and geographical distribution of ATM usage. For instance, by analyzing transaction patterns over time, the team can identify peak hours of ATM usage and anticipate potential congestion or downtime. They can also detect any irregularities in transaction patterns, such as a sudden increase in transaction failures or unusually high withdrawal amounts, which may indicate potential security breaches or technical issues.
Furthermore, BI tools equipped with geospatial analytics capabilities enable the team to visualize ATM locations on a map and assess the distribution of ATM usage across different regions. This insight can aid in strategic decisions regarding ATM placement, maintenance scheduling, fraud detection, and resource allocation to optimize service availability and enhance customer satisfaction.
Example 2: Assessment of the Bank’s Overall Financial Health
Let’s consider a scenario in a bank where risk analysts need to assess the overall financial health of the organization by integrating data from various sources. The data includes transaction records from the core banking system, customer information from a CRM system, market data from external financial markets, and economic indicators from online sources. With a few clicks, BI tools would be able to allow risk analysts to connect directly to diverse data sources, including the core banking system, CRM systems, external financial market APIs, and web-based economic indicators.
Example 3: Market Risk Analysis
Let’s assume a scenario where the risk management team of a bank uses a BI tool to link the stock market data to stock prices as part of its market risk analysis and gain insights into how market conditions can affect the bank’s investment portfolio. Using time series chart, the risk management team can display stock prices over time and observe trends, fluctuations, and correlations between stock prices and market conditions. This can help identify patterns in how market conditions impact the bank’s investment portfolio. To address risk of market volatility, the risk management team can use a candlestick chart which would provide a detailed view of stock price movements, including open, high, low, and close prices and would be especially helpful when the user needs to spot volatility.
Example 4: Creditworthiness Assessment and Suspicious Transaction Monitoring
Let’s envision a scenario where a bank offers a variety of credit products, including credit cards and personal loans. To manage credit risk effectively, the bank needs to continuously assess the creditworthiness of its customers. BI tools can be used to generate a dynamic dashboard that tracks customer credit behaviours and highlights potential areas of concern that may require attention. By integrating data from the bank’s customer relationship management (CRM) system, transaction records, and credit bureau data, the dashboard can provide real-time insights into customer credit usage patterns.
Additionally, integrating a customer segmentation model within business intelligence tools can further enhance the bank’s credit risk management strategy. By leveraging such models, the bank can delve deeper into customer spending and financial habits, categorizing customers into distinct segments based on their behavior. This segmentation allows for a more personalized approach to credit product offerings as well as management of credit risk.
Automated alerting system of BI tools can be also configured to trigger alerts based on pre-defined criteria. If a customer’s credit card spending suddenly spikes beyond their historical patterns or if there are missed loan payments, the respective tool can promptly notify the bank’s credit risk team. These alerts can provide an early warning system for potential credit risks. For example, if a customer’s credit score drops significantly, the credit risk team can be alerted to review the customer’s account and assess the need for credit limit adjustments or additional risk mitigation measures.
Since many modern BI tools offer advanced analytics capabilities, including the ability to integrate custom statistical models using statistical software or programming languages, the bank can also integrate a statistical model into its BI tool to analyze various factors and identify potential credit risks based on patterns in the data. For example, a statistical model might consider not only individual events like sudden spikes in spending but also the overall spending behavior of a customer compared to historical patterns, as well as other relevant variables such as credit score changes, economic indicators, and demographic information.
Example 5: Credit Card Transaction Monitoring
Imagine a scenario where a bank’s risk management team is tasked with monitoring credit card transactions for potentially fraudulent activity. Using a BI tool with real-time monitoring capabilities, they set up a dashboard to track transaction volumes, spending patterns, and geographical locations of card usage in real-time.
As transactions occur, the BI tool analyzes the data instantly, flagging any suspicious activities such as unusually large purchases, transactions from unfamiliar locations, or a sudden increase in declined transactions. Automated alerts are triggered, notifying the risk management team of potential fraud attempts.
For example, if a credit card is used for multiple high-value purchases within a short period, the BI tool immediately raises an alert, prompting the team to investigate further. By swiftly identifying and responding to suspicious transactions in real-time, the bank can prevent fraudulent activities, minimize financial losses, and safeguard its customers’ assets.
Responding to Complex Challenges
In today’s banking sector, characterized by ever-evolving regulatory landscapes, volatile market conditions, and increasing customer expectations, the need for robust risk analytics capabilities has never been more pronounced. Business Intelligence tools have emerged as a vital tool, offering comprehensive solutions to the complex challenges faced by financial institutions, particularly by banks.
By harnessing the power of BI tools, banks can seamlessly integrate data from diverse sources, enabling a holistic view of their operations, customer interactions, and market dynamics. This integrated approach streamlines risk assessment processes, reduces manual errors, and enhances the accuracy of decision-making. Moreover, BI tools empower risk analysts to transform raw data into actionable insights through advanced visualization techniques, such as customizable charts, graphs, and dashboards.
Practical examples from the banking industry illustrate the tangible benefits of leveraging BI tools in risk analytics for the financial sector. From assessing overall financial health to monitoring credit behaviours and market trends, BI tools provide strong monitoring capabilities that enable proactive risk management. By identifying required insights and potential risks in a more structured manner and early on, banks can mitigate losses, optimize resource allocation, and maintain regulatory compliance effectively.
As the financial sector’s digital transformation continues to progress, the role of BI tools as catalysts in risk management and data analytics becomes increasingly pivotal. Institutions that embrace these technologies gain a competitive edge, fostering agility, innovation, and sustainable growth. By leveraging BI tools, banks can navigate uncertainties with confidence, making more informed decisions that drive long-term success and resilience in an ever-changing landscape. BI tools not only revolutionize risk analytics but also pave the way for a more secure and prosperous future for the banking industry.
Mert Ozbilgin works as a senior regulatory advisor in a bank in Malta.