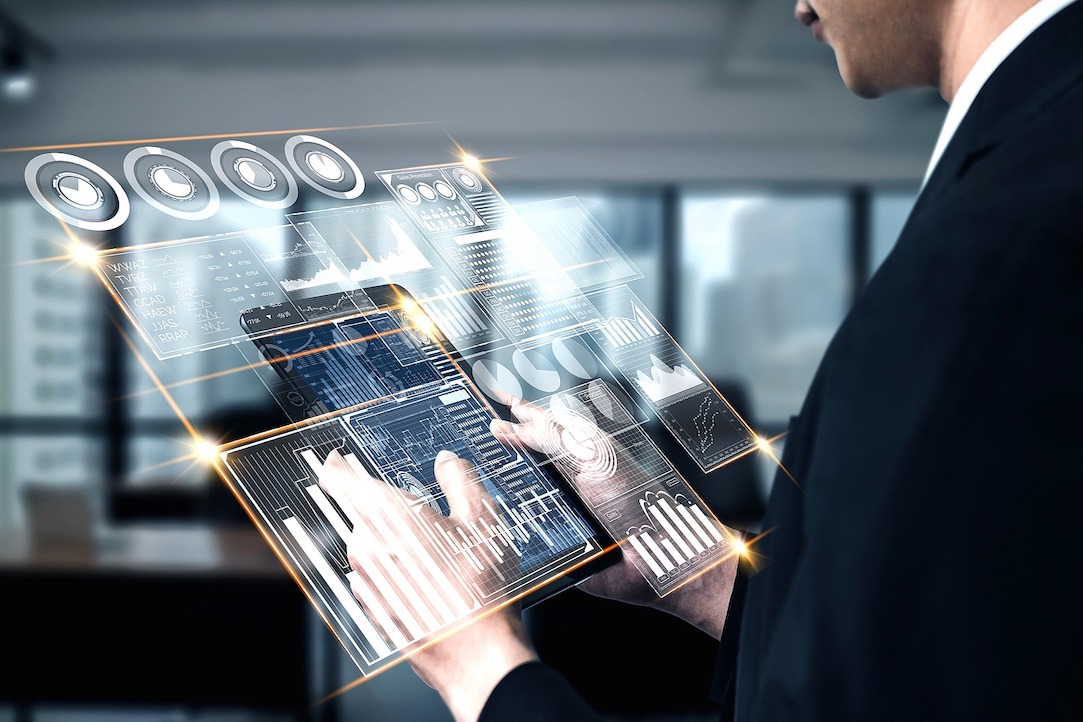
It’s been more than a year since generative artificial intelligence (GenAI) burst onto the scene with mainstream appeal. These new tools have been presented by some as an unstoppable force, upending industries and changing the way people work.
Generative AI can have an immense upside for organizations across many corporate functions, but as with any emerging technology, there are risks that internal auditors and governance professionals should consider. They must assess how those risks impact business processes and related controls. Moreover, internal auditors can prepare to understand how emerging AI tools can improve the internal audit function, offering not a replacement for auditors’ skills, but rather an opportunity to enhance them.
Amid the hype of AI, as internal auditors prepare their organizations for the opportunities and risks it presents, they may need to demystify AI and its underlying algorithms to understand how it works, and learn its capabilities, limitations, and potential impacts to the internal audit profession.
By understanding the basics, internal auditors may see past the myths of the technology, clearly understand the risks and potential impacts to business processes and related controls, as well as use AI more productively with a critical eye.
At its core, AI is an ensemble of algorithms and techniques designed to process information and make decisions in ways that mimic human intelligence. Many processes and technologies already used by finance departments and other corporate functions are powered by forms of AI, machine learning, and algorithms. For example, rather than manually approving invoices, an organization may use automated decision systems, a subset of algorithms, to provide approvals based on predetermined thresholds, vendor lists, and other criteria.
Algorithm Intelligence, Complexity, and Type
When evaluating potential risks of AI, it’s important for internal auditors to understand and classify the underlying algorithms by their intelligence, complexity, and type.
- Intelligence: In the context of algorithms, intelligence can be defined as “adaptation with insufficient knowledge and resources.” Low-intelligence algorithms lack the ability to adapt. Algorithms with predefined rules typically cannot deviate or expand upon these rules. These are typically lower risk. On the other hand, high-intelligence algorithms dynamically solve problems even as input data deviates from the type of data used for model training. However, high-intelligence algorithms can present greater risk and can serve as “black box AI,” where the algorithms reach conclusions or decisions without providing any explanations as to how they were made.
- Complexity: Algorithm complexity “refers to the technical sophistication or quantity of its components or elements.” For example, a simple decision tree with a binary output would be classified as non-complex. What’s important to understand is that as the complexity of the algorithm increases, so often does the level of risk.
- Type: The type of algorithm will vary depending on the problem it is designed to solve. When identifying and assessing risks associated with algorithmic outputs, internal auditors may consider whether the business has selected an algorithm that is well suited to its task. Understanding the problem, the goal, and the related algorithm type is important to any assessment of an algorithm and its risks.
AI is a Tool for Enhancement, not Replacement
In addition to mitigating organizational risk associated with the adoption of AI tools, internal audit professionals may seek to understand how emerging AI applications can enhance their skills. Despite what sensationalists may have you believe, AI is not a sentient entity, and it is not a replacement for human skills and judgement. Just as understanding AI’s underlying algorithms positions external audit professionals to better understand risk, this knowledge can empower effective use by internal auditors and allow them to critically evaluate their outputs.
The fear that AI will replace human judgment and diligence stems from a misunderstanding of its capabilities. AI excels at tasks requiring pattern recognition, data analysis, and automation. However, it does not have the same critical thinking, creativity, and ethical decision-making skills as humans. AI is not a replacement for internal auditors; it’s an augmentation tool that can enhance skills and improve effectiveness. AI offers several potentially valuable applications for internal auditors:
- Data Analysis: AI can analyze vast amounts of data and identify anomalies or trends that could be difficult for humans to detect. This can enhance risk assessments and fraud detection capabilities.
- Automation: AI can automate repetitive tasks such as data collection and report generation, freeing up time to focus on higher-level analysis and critical thinking.
- Continuous Monitoring: AI-powered tools can continuously monitor internal controls and identify potential issues in real-time, allowing for proactive risk mitigation.
Using AI for Better Decision Making
By understanding AI’s capabilities and limitations, internal auditors can strengthen their contribution to organizational governance and risk management. And by using AI with greater clarity, internal auditors can improve their skillset making them more effective with the capacity for stronger decision making. This can have immediate and far-reaching benefits for the organization and internal auditors themselves.
Similarly, as AI proliferates, internal auditors may also have a responsibility to ensure that AI systems are implemented and used responsibly across their organizations. By thinking carefully about the benefits, pitfalls and opportunities with AI systems, internal auditors can improve their work.
Brian Cassidy is Audit & Assurance AI Leader at Deloitte & Touche LLP. Ryan Hittner is Artificial Intelligence and Algorithmic Practice Co-leader, and Audit & Assurance Principal at Deloitte & Touche LLP.