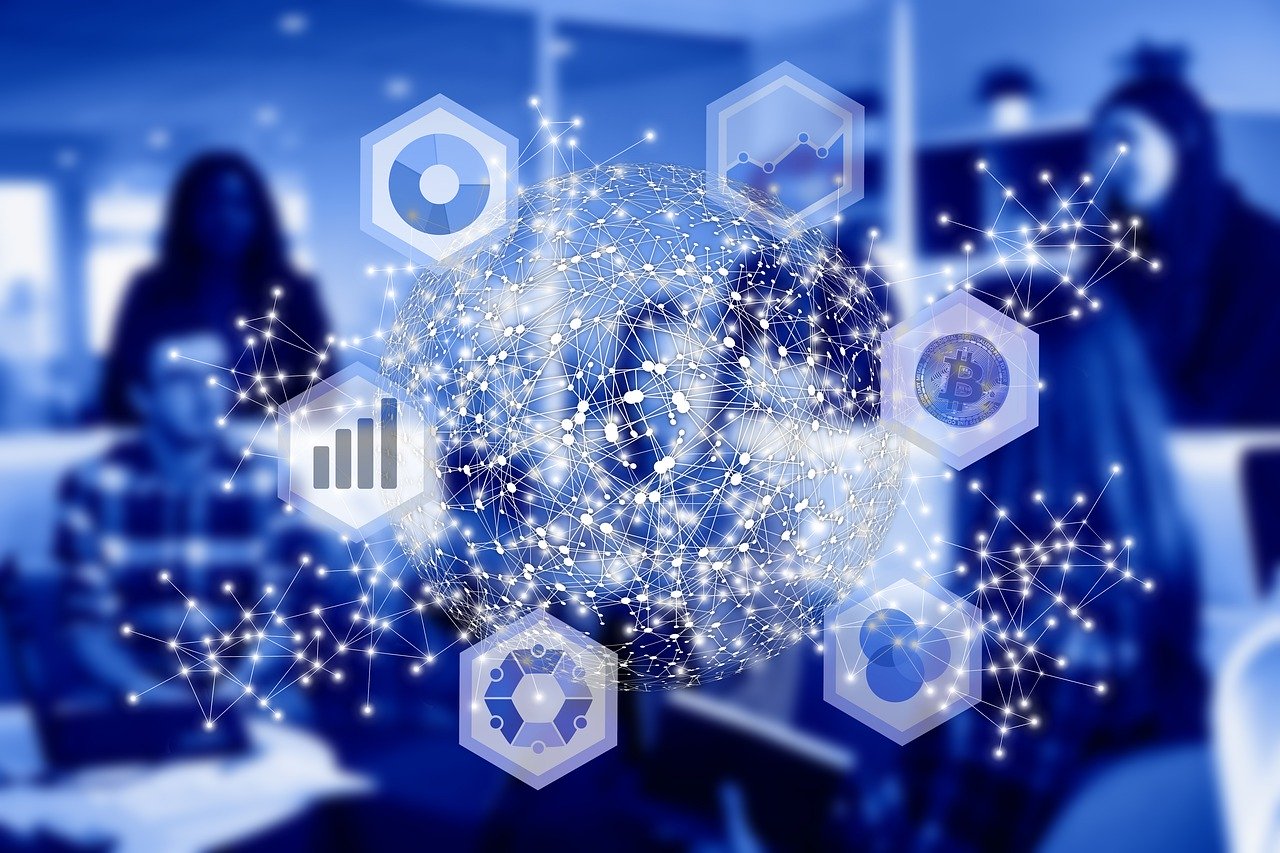
By now, most internal audit shops have at least dipped a toe in the waters of data analytics.
They are using data analysis techniques to find outliers in expense reports, to look for suppliers that have suspicious attributes or are at high risk for bribery, and for testing controls and identifying red flags.
According to recent PwC State of Internal Audit report, 82 percent of internal audit functions surveyed say they have increased their investment in data mining and data analytics to facilitate monitoring of key trends and to support continuous auditing.
As that report also states, however: “Many functions are finding their analytics programs stalled and in need of a jump start.” Indeed, many internal audit shops find it difficult to take their data analytics programs to the next level. They want to embed advanced analytics into everything they do. They want analytics to inform risk assessments, audit planning, and to be used in just about every audit. They also want to move into predictive analytics, which relies on data mining, predictive modeling, and machine learning, to analyze current and historical facts to make predictions about unknown events.
So what’s holding them back? For one, internal audit functions are finding it hard to hire the talent they need with data analysis backgrounds and capabilities. Most chief audit executives will tell you that finding audit candidates with data analytics skills is never easy.
Another issue is time. Most internal audit departments are stretched thin and don’t have the time to devote to coming up to speed on new technologies, including data analytics, even if it would save time in the long term. Technology is moving so fast that some CAEs decide they can’t keep up with it all and just throw up their hands.
According to Michael Smith, U.S. intelligent automation and solution lead for internal audit at KPMG, at companies where internal audit is behind on data analytics, the problems often lie higher up in the organization. “At organizations where internal audit isn’t particularly tech-savvy, it’s usually some combination of the lack of vision among company leaders and the positioning of the internal audit function.” He says they haven’t been empowered to go and create their own innovation.
Most internal audit departments, however, recognize the importance of initiating a more robust data analytics program. According to a study by internal audit consulting firm Protiviti, among internal audit teams not currently using advanced analytics, 19 percent plan to start using the tools in the next year, and another 47 percent plan to do so in the next two years.
So how can companies leverage data analytics to take their internal audit functions to the next level? Here are seven steps that can help supercharge a data analytics program.
Seven Ways to Improve Data Analytics Maturity
1. Demonstrate the potential. Provide even modest demonstrations of analytics capabilities that can win over skeptics and build support in the organization. When senior executives see the potential of what advanced data analytics can do, they will become more likely to provide increased budgets to fund the effort and managers may become less possessive of the data, which can be a big barrier to getting a data analytics program to the next level.
2. Name a data analytics champion. Internal audit functions with one or more dedicated analytics champions and dedicated analytics functions in place “deliver more value, experience higher demand for their analytics services, and obtain better access to higher-quality data,” states the Protiviti report. Having champions could help organizations to bridge the gap between the analytics function and operational auditors. It also encourages the use of analytics, including basic usage by the whole team. This dedicated leader can be invaluable in finding ways that analytics can improve audits or find more efficient ways of conducting them.
3. Get board and management support for data sharing. One of the biggest barriers to improving a data analytics program is getting access to quality data. Business and function owners can be particularly protective of their data and may not provide access or will guard parts of the best data sets. CAEs should explore avenues to expand internal audit’s access to quality data. That may include appealing to senior executives and even the board to push the importance of providing access to data throughout the organization. A mandate from the CEO can do wonders to loosen the tight grip some process owners maintain on their data.
4. Don’t get caught up on data analytics tools. Some internal audit functions get sidetracked on what data analytics package to select. Making that decision too early can harm a data analytics initiative. Placing too much focus on learning the tools can intimidate internal auditors and keep them from advancing. Most data analytics initiatives, experts say, start with something as simple as Microsoft Excel and move on when it becomes limiting to what auditors want to accomplish. There’s no reason to increase the complexity with advanced tools before your team has a good understanding of the data and what it can tell you.
5. Think creatively about data sources. The answers you seek may not lie in the data that is right in front of you. Data analytics practitioners must identify new data sources, both internal and external, that can enhance internal audit’s view of risk across the organization. This can ensure that the organization will be able to supplement data analytics procedures with a supply of quality data. Getting benchmark data from sources that aggregate it, for example, can provide a good baseline and comparison for analyzing the internal data.
6. Get stakeholder input. CAEs should seek ways to increase the level of input stakeholders provide when building and using data analytics models and continuous auditing tools. Process owners have the best understanding of the data and can be vital in helping to determine what data should be monitored. While many different stakeholders have important insights to help determine areas of focus, it is critical that the effort is focused on building tools that internal audit can leverage to monitor risk in the business.
7. Measure and report results. Finally, CAEs can implement steps to measure the success of their data analytics efforts and report success and value to management and other top stakeholders. Internal audit groups that successfully demonstrate tangible value are the ones that make a stronger business case for increased budgets and resources dedicated to data analytics. In the process, this can also boost the internal audit function’s reputation.
Pursuing these areas can help get a data analytics program off the ground or help move a program that is in the infant stage along the curve. Remember, starting small is better than not starting at all, since it won’t be long before small successes provide momentum to advance the program. Experiment, think creatively, and don’t be afraid to make mistakes. Internal audit shops that pursue data analytics without fear will soon be expanding their capabilities and unlocking the powerful potential of what it can do.
Joseph McCafferty is editor & publisher of Internal Audit 360°.